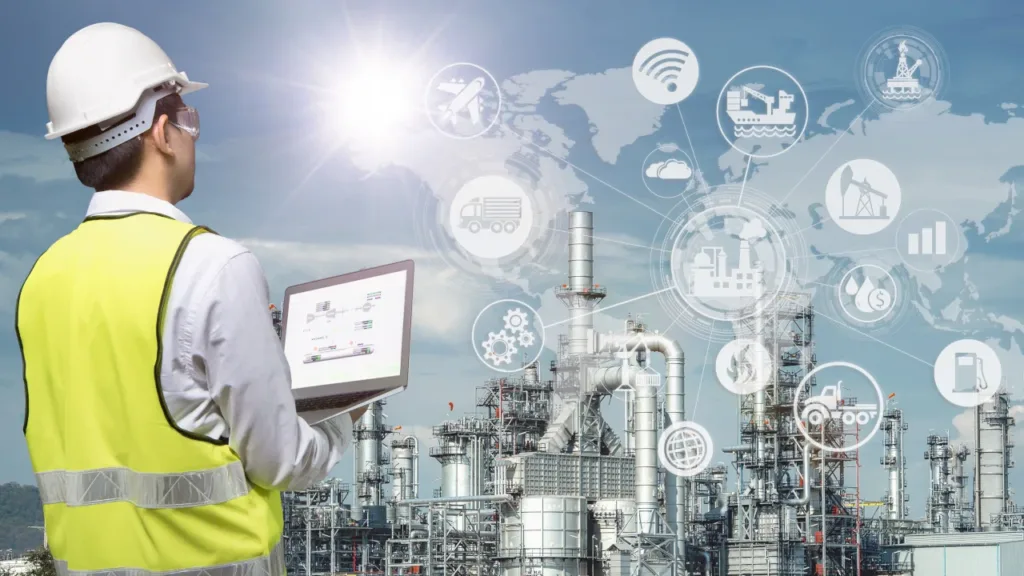
The Impact of AI and Big Data: Artificial Intelligence (AI) and Big Data are revolutionizing the oil and gas industry. These powerful technologies are reshaping everything from exploration and production to maintenance and environmental management. Discover how AI-driven analytics improve decision-making, enhance operational efficiency, and reduce costs. Learn about the role of predictive maintenance in preventing equipment failures and minimizing downtime, and how AI helps reduce the industry’s environmental impact. Join us as we delve into the profound changes AI and Big Data are bringing to one of the world’s most critical industries.
Introduction to AI and Big Data in the Oil and Gas Industry
The oil and gas industry has consistently been at the forefront of adopting innovative technologies to enhance its operations. From the initial transition to digital data logging in the 1980s to the implementation of more advanced software systems in the subsequent decades, the sector has continually sought technological enhancements to optimize processes. Today, the integration of Artificial Intelligence (AI) and Big Data is poised to revolutionize the industry even further.
One of the primary drivers for the adoption of AI and Big Data in the oil and gas sector is the need to address complex challenges related to exploration, production, and operational efficiency. Traditional methods often fall short in managing the vast and diverse datasets generated by oil exploration and extraction activities. AI and Big Data offer advanced analytics and predictive modeling capabilities, enabling industry professionals to make more informed decisions and significantly reduce operational costs.
AI algorithms can process enormous volumes of data at unprecedented speeds, uncovering patterns and insights that would be impossible for humans to detect manually. This capability is crucial in areas such as predictive maintenance, where AI can forecast equipment failures before they occur, minimizing downtime and potential financial losses. Similarly, Big Data analytics facilitate more efficient reservoir management and optimization of extraction processes, ensuring that resources are utilized to their full potential.
Historical technological advances laid the groundwork for today’s adoption of AI and Big Data, but the rapidly evolving digital landscape presents new opportunities. Machine learning models, for instance, are being increasingly employed to enhance seismic data interpretation, thus improving the accuracy of locating oil reserves. The growing complexity of operational environments and the escalating demand for energy underscore the pressing need for these innovations.
In summary, the incorporation of AI and Big Data in the oil and gas industry not only addresses critical pain points but also sets the stage for a more efficient and sustainable future. This convergence of technology is becoming indispensable, heralding a new era of digital transformation in the sector.
Data Collection and Analysis in Oil and Gas Operations
Data collection and analysis play a pivotal role in optimizing operations within the oil and gas industry. The types of data collected are diverse, encompassing geological, seismic, and operational data. Geological data, which includes rock formations, mineral compositions, and subsurface structures, is critical for identifying potential drilling sites. Seismic data, obtained through technologies like 3D seismic imaging, helps create detailed subsurface maps, enhancing the accuracy of geological data and aiding in the detection of hydrocarbon reservoirs.
Operational data covers a broad spectrum from daily production metrics, equipment performance, to environmental monitoring. The integration of these varied datasets forms the foundation of robust data analysis systems in the sector. With the advent of Big Data technologies, the industry’s approach to managing and analyzing massive datasets has undergone a substantial transformation. Advanced storage solutions such as Hadoop Distributed File System (HDFS) and cloud services enable the handling and processing of petabytes of data, facilitating the extensive retention and rapid retrieval of complex data sets.
Real-time processing of data is of immense significance in oil and gas operations. Technologies such as Apache Kafka and Apache Spark allow for the continuous streaming and instant analysis of data, providing operators with immediate insights and enabling swift, informed decision-making. These real-time processing capabilities are crucial in scenarios such as monitoring drilling activities and managing pipeline operations to mitigate risks and enhance efficiency.
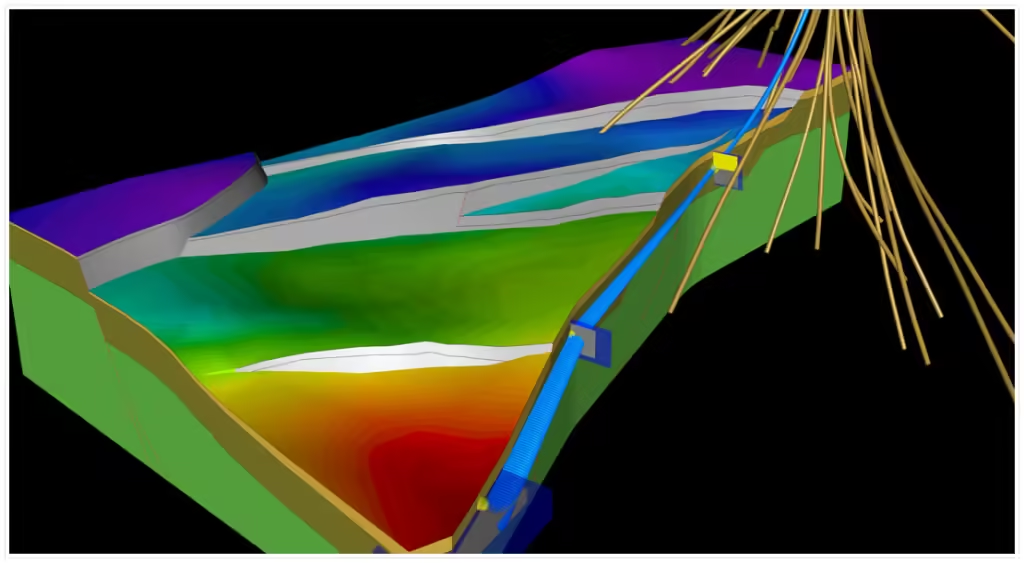
Several key tools and platforms are instrumental in the data analysis landscape of the oil and gas industry. Tools like Petrel for geological and geophysical data interpretation, PI System for operational data management, and OLAP cubes for multi-dimensional data analysis are widely utilized. These platforms support the integration and comprehensive analysis of varied data types, driving innovation and operational excellence within the sector.
AI-Powered Predictive Maintenance
Artificial Intelligence (AI) is revolutionizing the operational landscape of the oil and gas industry through its application in predictive maintenance. By leveraging advanced AI algorithms, companies are able to analyze vast amounts of data gathered from an array of sensors and equipment. This data-driven approach enables the prediction of equipment failures before they happen, thereby transforming maintenance strategies from reactive to proactive.
Predictive maintenance employs machine learning models and data analytics to monitor the health of machinery and infrastructure in real-time. These AI systems continuously learn from historical data patterns to forecast potential failures with remarkable accuracy. For instance, vibration sensors on drilling equipment, temperature sensors on pipelines, and pressure sensors on storage tanks collectively generate critical data that AI algorithms scrutinize to detect anomalies indicative of impending malfunctions.
The advantages of integrating AI-powered predictive maintenance are manifold. Firstly, it significantly reduces unplanned downtime, ensuring that operations run smoothly and efficiently. Secondly, it leads to substantial cost savings by minimizing emergency repairs and optimizing maintenance schedules. Thirdly, predictive maintenance enhances workplace safety by preventing catastrophic failures that could endanger personnel and the environment.
Several oil and gas companies have successfully implemented predictive maintenance systems, showcasing tangible benefits. For example, BP has integrated AI-driven predictive maintenance tools that have reportedly cut downtime by 20% and maintenance costs by 15%. Similarly, Shell’s utilization of AI has resulted in substantial improvements in equipment reliability and safety.
In conclusion, AI-powered predictive maintenance is proving to be a game-changer for oil and gas operations. By enabling the early detection of equipment issues, it not only drives operational efficiency and cost-effectiveness but also fortifies safety protocols, ensuring a more reliable and safer working environment.
Enhanced Exploration and Drilling through Machine Learning
Machine learning models are fundamentally transforming exploration and drilling activities in the oil and gas sector. By leveraging historical and real-time data, these models can pinpoint optimal drilling locations with unparalleled precision. Traditionally, identifying these locations involved extensive geological surveys and significant time investments. However, with machine learning, geological data, seismic readings, and well logs are analyzed to forecast where valuable resources are likely to be found.
Machine learning enhances the accuracy of predictions, minimizing the risk of dry wells and reducing the overall cost of exploration. Real-time data integration allows for continuous updates and refinements to the models, ensuring that drilling strategies remain aligned with the most current information available. Moreover, machine learning can predict drilling hazards and optimize equipment usage, thus improving operational efficiency.
The benefits extend beyond merely finding resources. Machine learning helps in refining reservoir models, which leads to more efficient extraction processes and better resource management. For instance, predictive maintenance facilitated by machine learning can foresee equipment failures before they occur, reducing downtime and associated costs. Thus, operational effectiveness and safety are significantly enhanced.
Several companies have already demonstrated the efficacy of machine learning in exploration and drilling. For example, Royal Dutch Shell has integrated machine learning into its exploration workflows, resulting in more accurate subsurface maps and improved drilling success rates. Similarly, BP has utilized machine learning algorithms to process seismic data and identify promising drilling sites, leading to faster decision-making and reduced operational expenditures.
The adoption of machine learning in these activities underscores a broader trend in the oil and gas industry towards digitalization and data-driven decision-making. This shift not only revolutionizes how companies explore and drill but also sets a precedent for future technological advancements in the field.
Optimizing Production with AI and Big Data
The integration of AI and Big Data is transforming the oil and gas industry, particularly in optimizing production processes. Leveraging data analytics, companies can now delve deeper into their operational data, extracting valuable insights that drive efficiency and maximize output. Through sophisticated AI algorithms, it is possible to continuously monitor and adjust production parameters in real-time, ensuring optimal performance across the board.
One of the primary advantages of utilizing AI in production optimization lies in its capability to analyze vast amounts of data swiftly and accurately. By processing data from various sensors and operational equipment, AI systems can detect patterns and anomalies that may signify potential inefficiencies or upcoming issues. This proactive approach allows for timely adjustments, minimizing downtime and mitigating the risk of costly disruptions.
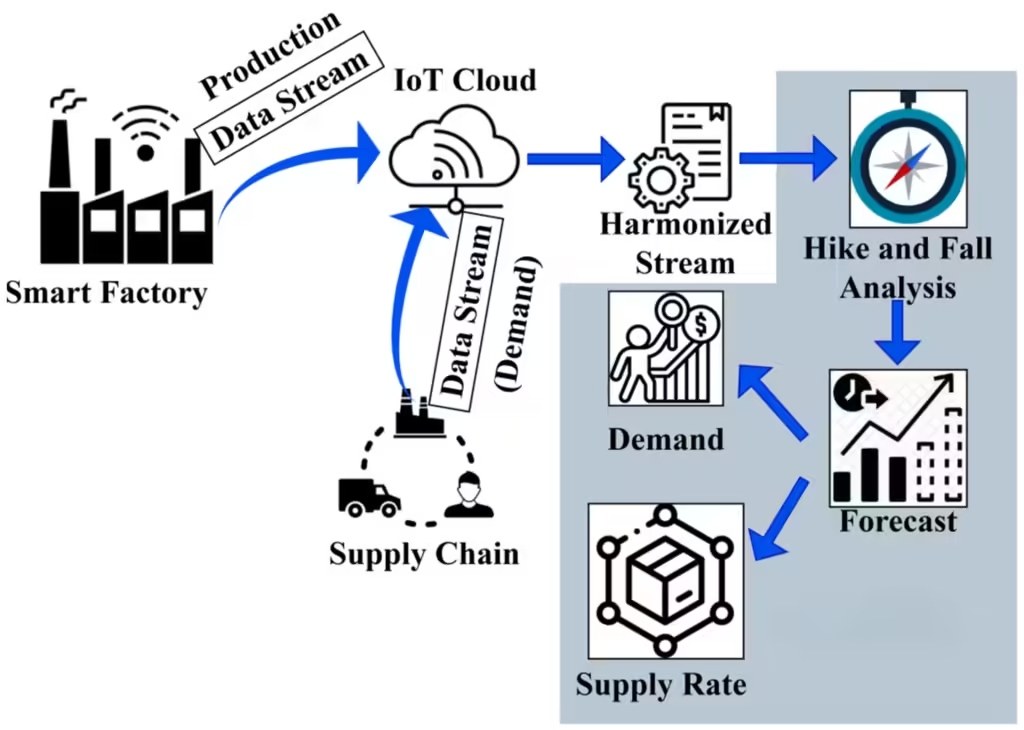
Predictive modeling and simulations play a crucial role in harnessing the full potential of these technologies. By creating detailed models of oil and gas operations, it becomes feasible to predict future outcomes based on historical data and current operational conditions. These simulations enable decision-makers to test different scenarios and strategies, leading to more informed and effective production planning. For example, Chevron has implemented predictive analytics to forecast equipment failures before they occur, significantly reducing maintenance costs and production delays.
Further, AI-driven technologies enhance the predictive capabilities by factoring in a wide range of variables that humans might overlook. Machine learning algorithms, in particular, learn from past data, continuously refining their predictions and adapting to new information. This iterative process results in ever-improving accuracy and reliability, driving continuous improvement in production efficiency.
In real-world applications, Shell has utilized AI and data analytics to optimize drilling operations. By analyzing geological data and drilling parameters, Shell’s AI systems have optimized drilling speeds and reduced non-productive time, yielding substantial cost savings and improved production outputs. Similarly, BP uses machine learning to analyze seismic data, improving the accuracy of oil reservoir estimations and enhancing extraction techniques.
Overall, the implementation of AI and Big Data in oil and gas production is not just a technological advancement but a strategic necessity. The ability to optimize production through advanced data analytics and AI is paving the way for more sustainable and profitable operations in this highly competitive industry.
AI and Big Data in Supply Chain Management
The integration of AI and Big Data in the supply chain management of the oil and gas industry represents a significant advancement in operational efficiency. These technologies are pivotal in transforming traditional supply chain processes by enabling precise demand forecasting, comprehensive inventory management, and seamless logistics operations. Consequently, oil and gas companies can achieve unprecedented levels of accuracy and efficiency in supply chain operations.
AI-driven demand forecasting relies on complex algorithms that analyze patterns and predict future trends, enabling companies to manage resources proactively. Through predictive analytics, oil and gas sectors can anticipate demand fluctuations, allowing them to adjust production schedules and inventory levels accordingly. This predictive capability reduces the risk of overproduction or stock shortages, leading to optimized inventory levels and cost savings.
Additionally, Big Data plays a crucial role in supply chain management by providing real-time insights into every aspect of the supply chain. Advanced sensors and IoT devices collect vast amounts of data that can be analyzed to monitor equipment performance, track shipment status, and identify potential bottlenecks. This data-driven approach ensures that logistic operations are streamlined, reducing delays and enhancing overall supply chain efficiency.
One prominent example of these technologies at work is Shell’s implementation of AI and Big Data strategies within their supply chain. By leveraging these technologies, Shell has optimized its inventory management, reducing excess stock and minimizing storage costs. Another example is BP’s use of advanced analytics to enhance its supply chain logistics, which has led to more efficient routing of shipments and a marked decrease in transportation costs.
The benefits of applying AI and Big Data in supply chain management extend beyond cost savings. Enhanced decision-making capabilities allow companies to respond swiftly to market changes and operational challenges, maintaining a competitive edge in the industry. As AI and Big Data technologies continue to evolve, their role in refining supply chain processes will undoubtedly become even more integral, further driving progress in the oil and gas sector.
Environmental and Safety Improvements
AI and Big Data technologies are pivotal in driving substantial environmental and safety improvements within the oil and gas sector. By leveraging predictive analytics and real-time monitoring systems, the industry can address environmental concerns and bolster safety protocols with heightened precision and efficiency.
The application of predictive analytics is remarkable in its ability to foresee potential issues before they escalate. For instance, real-time data collection and analysis enable the early detection of equipment malfunctions, reducing the likelihood of leaks that could cause environmental damage. This preemptive approach fosters a safer operational environment while diminishing the impact on surrounding ecosystems.
Leak detection systems have significantly evolved through the integration of AI. Traditional methods, often reliant on periodic inspections or employee observations, are now supplemented or replaced by AI-driven solutions. These sophisticated systems continuously monitor pipelines and infrastructure, identifying and addressing leaks instantaneously. This not only minimizes environmental hazards but also ensures regulatory compliance, averting any legal repercussions.
Furthermore, AI’s role in emission monitoring cannot be understated. Advanced algorithms analyze data from various sensors, providing real-time insights into emissions output. This enables operators to make timely adjustments, ensuring that emissions remain within permissible limits and mitigating any adverse environmental impacts. Such technologies are crucial in the industry’s efforts to reduce its carbon footprint and comply with stringent environmental regulations.
Risk assessment is another area where Big Data shines. AI systems evaluate vast amounts of historical and real-time data to predict potential safety hazards. These insights empower companies to implement proactive measures, safeguarding their workforce and the environment. For example, Chevron’s use of AI-enhanced risk assessment tools has led to a 30% reduction in safety incidents, underscoring the tangible benefits of these technologies.
Several case studies reinforce the efficacy of AI and Big Data in environmental protection and worker safety. BP’s deployment of AI for real-time monitoring has led to a 40% decrease in environmental incidents, showcasing a clear advantage in utilizing these technologies. Similarly, Shell’s implementation of predictive maintenance has resulted in a 25% drop in operational disruptions, translating to a safer workplace and reduced environmental impact.
In summary, the integration of AI and Big Data within the oil and gas industry marks a significant stride towards enhanced environmental stewardship and safety. Through the advanced capabilities of predictive analytics and real-time monitoring, the industry is better equipped to navigate the challenges of environmental and safety management.
Future Trends and Challenges
The integration of AI and Big Data into oil and gas operations is anticipated to drive significant advancements in the sector. Future trends indicate a deeper embedding of AI-driven analytics, machine learning models, and Internet of Things (IoT) devices throughout the value chain. Innovations such as digital twins and predictive maintenance are on the horizon, harnessing vast datasets and real-time monitoring to optimize asset management, reduce downtime, and enhance operational efficiency.
However, there are several challenges the industry must address to fully leverage these technologies. One major concern is data privacy. As the volume of data collected grows exponentially, safeguarding sensitive information and ensuring compliance with data protection regulations becomes paramount. Similarly, cybersecurity is a critical issue, given the increasing threat of cyber-attacks on digital infrastructures. It is essential for oil and gas companies to implement robust security measures and continuously adapt to evolving threats.
The demand for skilled personnel adept in both traditional oil and gas operations and advanced AI and data analytics is another challenge. The industry needs to invest in training and development programs to cultivate a workforce capable of navigating this technological shift. Educational institutions and industry stakeholders must collaborate to create curricula that bridge the gap between traditional engineering principles and modern data science skills.
Despite these challenges, the transformative potential of AI and Big Data in the oil and gas industry is undeniable. By leveraging these technologies, companies can achieve greater precision in exploration, enhanced efficiency in production, and superior safety across operations. To realize these benefits, the industry must prioritize investments in technology, cybersecurity, and workforce development, ensuring a comprehensive approach to modernization.